Join the 2024 Call for Code Global Challenge!
Be a part of a new era in responsible AI. Use your skills to improve equitable access to essential resources and opportunities for all people. You could win prizes and help show the world that AI can be trusted and used responsibly.
How will you answer the call?Get started Watch video
How to get involved
We’re calling all university students, developers, and problem solvers to join Call for Code. From the annual Global Challenge to additional events throughout the year, as well as ongoing open source projects, there are many ways to get involved. Participate in the ways that are right for you and build skills while making meaningful change.
Real code helping real people
Through Call for Code, top solutions are actively supported to bring the technology into communities in need, working with partners like the United Nations and the Linux Foundation. Deployments are underway across the globe.
See the top Call for Code solutions now being deployed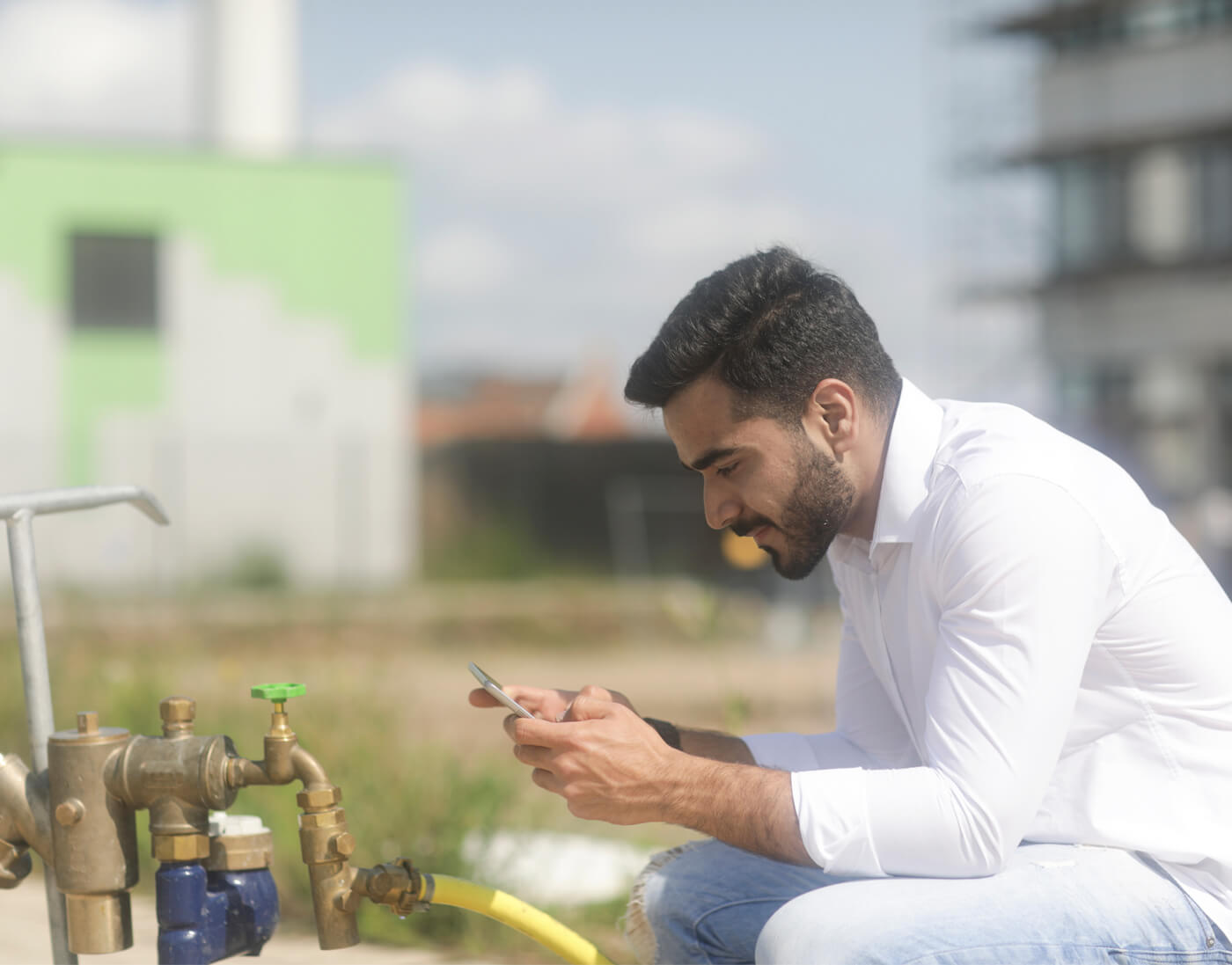
Partner with us
Call for Code has the year-round support of enterprises, experts, humanitarian and international organizations, charitable partners, celebrities, and more. There are many ways you can partner with Call for Code, whether it’s providing expertise, problem solving and technical skills, deploying or adopting projects, or offering financial support.
Be a Call for Code partner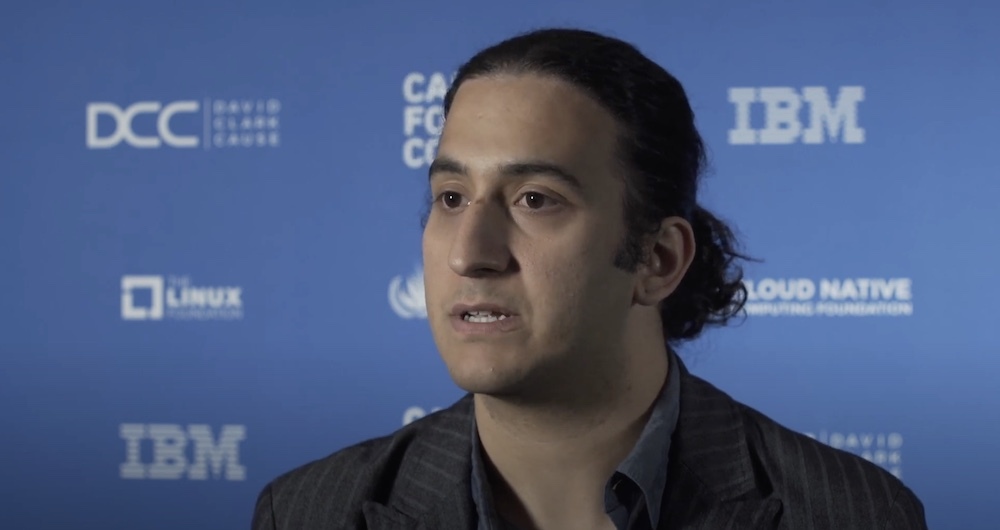
Share your support
Join a community of developers and innovators using open source technology to take on the world’s greatest issues. Challenge your friends, family, and colleagues to do the same by clicking a social network and making a personal post.
Share on your favorite platform
Twitter Facebook LinkedIn