Call for Code® for Racial Justice
Your skills can help make a difference in the fight for racial justice. Join
open source projects that help rewrite the wrong and combat racism, wherever it occurs.
Black
lives matter. Period.
Learn about the projects
With a focus on law and order, the electoral process, and policy-making, these projects were designed to help
combat racism and promote racial justice. We are inviting people across the globe to channel their
frustrations about systemic racism in a tangible way by getting involved with these projects to make an impact
in under-served and underrepresented communities.
Take a stand against racism and start contributing to the project of your choice. Explore the topics, learn
about the open source projects, and use the solution starters to understand where you can make the greatest
difference. Issues can be created and pull requests are made within the project GitHub repos themselves.
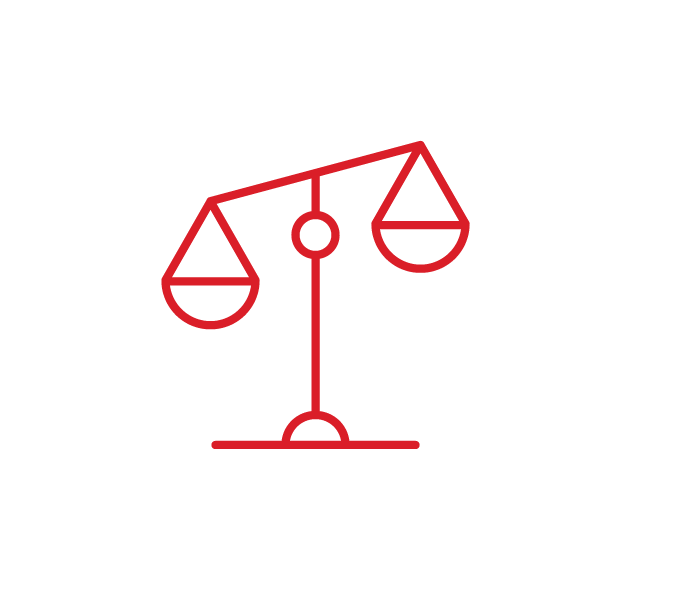
Police & Judicial Reform and Accountability
From community policing to sentencing and incarceration, data and technology has a role to play in the fight for racial equality.
Open Sentencing
To help public defenders better serve their clients and make a stronger case, Open Sentencing shows racial bias in data such as demographics.
Incident Accuracy Reporting System (IARS)
This platform allows witnesses and victims to corroborate evidence or provide additional information from multiple sources against an official police report.
Fair Change
This platform helps record and catalog evidence of potentially racially charged incidents to help enable transparency, reeducation and reform as a matter of public interest and safety.
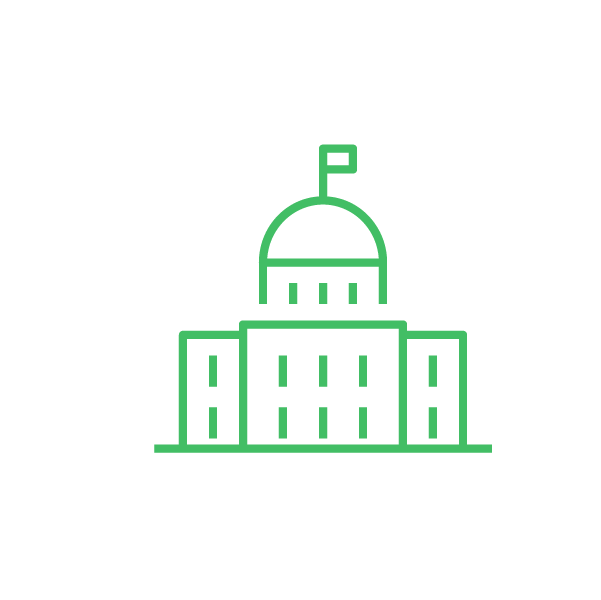
Policy & Legislation Reform
Policy and legislation needs more inclusion from the communities it impacts. This requires better visibility into the creation process and more opportunities for feedback.
Truth Loop
This app helps communities simply understand the policies, regulations, and legislation that will impact them the most.
Five Fifths Voter
This web app empowers minorities to exercise their right to vote and ensures their voice is heard by determining optimal voting strategies and limiting suppression issues.
Legit-Info
Local legislation can have significant impacts on areas as far-reaching as jobs, the environment, and safety. Legit-Info helps individuals understand the legislation that shapes their lives.

Diverse Representation
Bias and misrepresentation are commonplace throughout society and business. These projects serve to address these issues and strive for representation at every level.
TakeTwo
Bias in digital content such as news articles, blogs and even technical documentation can lead to overt or subtle racial discrimination. This application uses AI to identify bias in content using an iterative learning approach.
Share your support
Take a stand and join a community of developers, innovators, and social justice advocates to evolve or leverage the Call for Code for Racial Justice open source solutions. Challenge your friends, family, and colleagues to do the same.
Instructions:
1. Select the social platform to share your commitment. Make the post personal to you, and be sure to include the hashtag #CallforCodeforRacialJustice.
For example: “I am standing up against racism with #CallforCodeforRacialJustice. Get involved.”
2. Share your post, and come back to this page to learn more about the solutions.
Click the links below
Twitter Facebook LinkedIn